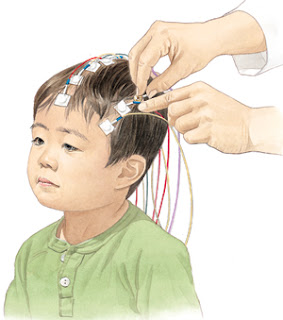
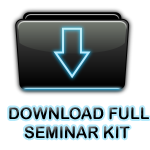
Derivatives of the EEG technique include evoked potentials (EP), which involves averaging the EEG activity time-locked to the presentation of a stimulus of some sort (visual, Somatosensory, or auditory). Event-related potentials refer to averaged EEG responses that are time-locked to more complex processing of stimuli; this technique is used in cognitive science, cognitive psychology, and psycho physiological research.
Epilepsy monitoring is typically done:
§ to distinguish epileptic seizures from other types of spells, such as psychogenic non-epileptic seizures, syncope (fainting), sub-cortical movement disorders and migraine variants.
§ to characterize seizures for the purposes of treatment
§ to localize the region of brain from which a seizure originates for work-up of possible seizure surgery
Additionally, EEG may be used to monitor certain procedures:
§ to monitor the depth of anesthesia
§ as an indirect indicator of cerebral perfusion in carotid endarterectomy
§ to monitor amobarbital effect during the Wada test
EEG can also be used in intensive care units for brain function monitoring:
§ to monitor for non-convulsive seizures/non-convulsive status epileptics
§ to monitor the effect of sedative/anesthesia in patients in medically induced coma (for treatment of refractory seizures or increased intracranial pressure)
§ to monitor for secondary brain damage in conditions such as subarachnoid hemorrhage (currently a research method)
If a patient with epilepsy is being considered for respective surgery, it is often necessary to localize the focus (source) of the epileptic brain activity with a resolution greater than what is provided by scalp EEG. This is because the cerebrospinal fluid, skull and scalp smear the electrical potentials recorded by scalp EEG. In these cases, neurosurgeons typically implant strips and grids of electrodes (or penetrating depth electrodes) under the dura mater, through either a craniotomy or a burr hole. The recording of these signals is referred to aselectrocorticography (ECoG), subdural EEG (sdEEG) or intracranial EEG (icEEG)--all terms for the same thing. The signal recorded from ECoG is on a different scale of activity than the brain activity recorded from scalp EEG. Low voltage, high frequency components that cannot be seen easily (or at all) in scalp EEG can be seen clearly in ECoG. Further, smaller electrodes (which cover a smaller parcel of brain surface) allow even lower voltage, faster components of brain activity to be seen. Some clinical sites record from penetrating microelectrodes.
Research use
EEG, and its derivative, ERPs, are used extensively in neuroscience, cognitive science, cognitive psychology, and psycho physiological research. Many techniques used in research contexts are not standardized sufficiently to be used in the clinical context.
A different method to study brain function is functional magnetic resonance imaging (fMRI). Some benefits of EEG compared to fMRI include:
§ Hardware costs are significantly lower for EEG sensors versus an fMRI machine
§ EEG sensors can be deployed into a wider variety of environments than can a bulky, immobile fMRI machine
§ EEG enables higher temporal resolution, on the order of milliseconds, rather than seconds
§ EEG is relatively tolerant of subject movement versus an fMRI (where the subject must remain completely still)
§ EEG is silent, which allows for better study of the responses to auditory stimuli
§ EEG does not aggravate claustrophobia
EEG also has some characteristics that compare favorably with behavioral testing:
§ EEG can detect covert processing (i.e., processing that does not require a response)
§ EEG can be used in subjects who are incapable of making a motor response
§ Some ERP components can be detected even when the subject is not attending to the stimuli
§ As compared with other reaction time paradigms, ERPs can elucidate stages of processing (rather than just the final end result)
2.Epilepsy:
Epilepsy is a common chronic neurological disorder characterized by recurrent unprovoked seizures.[1][2] These seizures are transient signs and/or symptoms of abnormal, excessive or synchronous neuronal activity in the brain.[3] About 50 million people worldwide have epilepsy, with almost 90% of these people being in developing countries.[4] Epilepsy is more likely to occur in young children, or people over the age of 65 years; however, it can occur at any time.[5] As a consequence of brain surgery, epileptic seizures may occur in recovering patients.
Epilepsy is usually controlled, but cannot be cured with medication, although surgery may be considered in difficult cases. However, over 30% of people with epilepsy do not have seizure control even with the best available medications.[6][7] Not all epilepsy syndromes are lifelong – some forms are confined to particular stages of childhood. Epilepsy should not be understood as a single disorder, but rather as syndromic with vastly divergent symptoms but all involving episodic abnormal electrical activity in the brain.
Epilepsies are classified in five ways:
1. By their first cause (or etiology).
2. By the observable manifestations of the seizures, known as semiology.
3. By the location in the brain where the seizures originate.
4. As a part of discrete, identifiable medical syndromes.
5. By the event that triggers the seizures, as in primary reading epilepsy or musicogenic epilepsy.
In 1981, the International League Against Epilepsy (ILAE) proposed a classification scheme for individual seizures that remains in common use.[8] This classification is based on observation (clinical and EEG) rather than the underlying path physiology or anatomy and is outlined later on in this article. In 1989, the ILAE proposed a classification scheme for epilepsies and epileptic syndromes.This can be broadly described as a two-axis scheme having the cause on one axis and the extent of localization within the brain on the other. Since 1997, the ILAE have been working on a new scheme that has five axes:
1. ictal phenomenon, (pertaining to an epileptic seizure)
2. seizure type,
3. syndrome,
4. etiology,
5. impairment.
Seizure types
Seizure types are organized firstly according to whether the source of the seizure within the brain is localized (partial or focal onset seizures) or distributed (generalized seizures). Partial seizures are further divided on the extent to which consciousness is affected. If it is unaffected, then it is a simple partial seizure; otherwise it is a complex partial (psychomotor) seizure. A partial seizure may spread within the brain - a process known as secondary generalization. Generalized seizures are divided according to the effect on the body but all involve loss of consciousness. These include absence (petit mal), myoclonic, clonic, tonic, tonic-clonic (grand mal) and atonic seizures.
Children may exhibit behaviors that are easily mistaken for epileptic seizures but are not caused by epilepsy. These include:
§ Inattentive staring
§ Benign shudders (among children younger than age 2, usually when they are tired or excited)
§ Self-gratification behaviors (nodding, rocking, head banging)
§ Conversion disorder (flailing and jerking of the head, often in response to severe personal stress such as physical abuse)
Conversion disorder can be distinguished from epilepsy because the episodes never occur during sleep and do not involve incontinence or self-injury.
3.Epileptic seizure
An epileptic seizure, occasionally referred to as a fit, is defined as a transient symptom of "abnormal excessive or synchronous neuronal activity in the brain". The outward effect can be as dramatic as a wild thrashing movement (tonic-clonic seizure) or as mild as a brief loss of awareness. It can manifest as an alteration in mental state, tonic or clonic movements, convulsions, and various other psychic symptoms (such as déjà vu or jamais vu). Sometimes it is not accompanied by convulsions but a full body "slump", where the person simply will lose control of their body and slump to the ground. The medical syndrome of recurrent, unprovoked seizures is termed epilepsy, but seizures can occur in people who do not have epilepsy.
About 4% of people will have an unprovoked seizure by the age of 80 and yet the chance of experiencing a second seizure is between 30% and 50%. Treatment may reduce the chance of a second one by as much as half.[3]Most single episode seizures are managed by primary care physicians (emergency or general practitioners), whereas investigation and management of ongoing epilepsy is usually by neurologists. Difficult-to-manage epilepsy may require consultation with an epileptologist, a neurologist with an interest in epilepsy.
4.Neural network:
Traditionally, the term neural network had been used to refer to a network or circuit of biological neurons the modern usage of the term often refers to artificial neural networks, which are composed of artificial neurons or nodes. Thus the term has two distinct usages:
1. Biological neural networks are made up of real biological neurons that are connected or functionally related in the peripheral nervous system or the central nervous system. In the field of neuroscience, they are often identified as groups of neurons that perform a specific physiological function in laboratory analysis.
2. Artificial neural networks are made up of interconnecting artificial neurons (programming constructs that mimic the properties of biological neurons). Artificial neural networks may either be used to gain an understanding of biological neural networks, or for solving artificial intelligence problems without necessarily creating a model of a real biological system. The real, biological nervous system is highly complex and includes some features that may seem superfluous based on an understanding of artificial networks.
This article focuses on the relationship between the two concepts; for detailed coverage of the two different concepts refer to the separate articles: Biological neural network and artificial neural network.
The brain, neural networks and computers
Neural networks, as used in artificial intelligence, have traditionally been viewed as simplified models of neural processing in the brain, even though the relation between this model and brain biological architecture is debated.
A subject of current research in theoretical neuroscience is the question surrounding the degree of complexity and the properties that individual neural elements should have to reproduce something resembling animal intelligence.
Historically, computers evolved from the von Neumann architecture, which is based on sequential processing and execution of explicit instructions. On the other hand, the origins of neural networks are based on efforts to model information processing in biological systems, which may rely largely on parallel processing as well as implicit instructions based on recognition of patterns of 'sensory' input from external sources. In other words, at its very heart a neural network is a complex statistical processor (as opposed to being tasked to sequentially process and execute).
Neural coding is concerned with how sensory and other information is represented in the brain by neurons. The main goal of studying neural coding is to characterize the relationship between the stimulus and the individual or ensemble neuronal responses and the relationship among electrical activity of the neurons in the ensemble. It is thought that neurons can encode both digital and analog information.
5.Artificial neural network (ANN)
An artificial neural network (ANN), usually called "neural network" (NN), is a mathematical model or computational model that is inspired by the structure and/or functional aspects of biological neural networks. It consists of an interconnected group of artificial neurons and processes information using a connectionist approach to computation. In most cases an ANN is an adaptive system that changes its structure based on external or internal information that flows through the network during the learning phase. Modern neural networks are non-linear statistical data modeling tools. They are usually used to model complex relationships between inputs and outputs or to find patterns in data.
Real life applications
The tasks to which artificial neural networks are applied tend to fall within the following broad categories:
§ Function approximation, or regression analysis, including time series prediction, fitness approximation and modeling.
§ Classification, including pattern and sequence recognition, novelty detection and sequential decision making.
§ Data processing, including filtering, clustering, blind source separation and compression.
§ Robotics, including directing manipulators, Computer numerical control.
Application areas include system identification and control (vehicle control, process control), quantum chemistry, game-playing and decision making (backgammon, chess, racing), pattern recognition (radar systems, face identification, object recognition and more), sequence recognition (gesture, speech, handwritten text recognition), medical diagnosis, financial applications (automated trading systems),data mining (or knowledge discovery in databases, "KDD"), visualization and e-mail spam filtering.
Fully recurrent network
This is the basic architecture developed in the 1980s: a network of neuron-like units, each with a directed connection to every other unit. Each unit has a time-varying real-valued activation. Each connection has a modifiable real-valued weight. Some of the nodes are called input nodes, some output nodes, the rest hidden nodes. Most architecture below are special cases.
For supervised learning in discrete time settings, training sequences of real-valued input vectors become sequences of activations of the input nodes, one input vector at a time. At any given time step, each non-input unit computes its current activation as a nonlinear function of the weighted sum of the activations of all units from which it receives connections. There may be teacher-given target activations for some of the output units at certain time steps. For example, if the input sequence is a speech signal corresponding to a spoken digit, the final target output at the end of the sequence may be a label classifying the digit. For each sequence, its error is the sum of the deviations of all target signals from the corresponding activations computed by the network. For a training set of numerous sequences, the total error is the sum of the errors of all individual sequences. Algorithms for minimizing this error are mentioned in the section on training algorithms below.
In reinforcement learning settings, there is no teacher providing target signals for the RNN, instead a fitness function or reward function is occasionally used to evaluate the RNN's performance, which is influencing its input stream through output units connected to actuators affecting the environment. Again, compare the section on training algorithms below.
This figure depicts such a decomposition of
, with dependencies between variables indicated by arrows. These can be interpreted in two ways.

The first view is the functional view: the input
is transformed into a 3-dimensional vector
, which is then transformed into a 2-dimensional vector
, which is finally transformed into
. This view is most commonly encountered in the context of optimization.




The second view is the probabilistic view: the random variable
depends upon the random variable
, which depends upon
, which depends upon the random variable
. This view is most commonly encountered in the context of graphical models.




The two views are largely equivalent. In either case, for this particular network architecture, the components of individual layers are independent of each other (e.g., the components of
are independent of each other given their input
). This naturally enables a degree of parallelism in the implementation.


Networks such as the previous one are commonly called feedforward, because their graph is a directed acyclic graph. Networks with cycles are commonly called recurrent. Such networks are commonly depicted in the manner shown at the top of the figure, where
is shown as being dependent upon itself. However, there is an implied temporal dependence which is not shown.

See also: Graphical models
No comments:
Post a Comment
leave your opinion