Computing in its purest form, has changed hands multiple times. First, from near the beginning mainframes were predicted to be the future of computing. Indeed mainframes and large scale machines were built and used, and in some circumstances are used similarly today. The trend, however, turned from bigger and more expensive, to smaller and more affordable commodity PCs and servers.
Need for large data processing
COMPARISON WITH OTHER SYSTEMS
SUBPROJECTS
Core
Avro
Mapreduce
HDFS
Pig
THE HADOOP APPROACH
Data distribution
Most of our data is stored on local networks with servers that may be clustered and sharing storage. This approach has had time to be developed into stable architecture, and provide decent redundancy when deployed right. A newer emerging technology, cloud computing, has shown up demanding attention and quickly is changing the direction of the technology landscape. Whether it is Google’s unique and scalable Google File System, or Amazon’s robust Amazon S3 cloud storage model, it is clear that cloud computing has arrived with much to be gleaned from.
Cloud computing is a style of computing in which dynamically scalable and often virtualize resources are provided as a service over the Internet. Users need not have knowledge of, expertise in, or control over the technology infrastructure in the "cloud" that supports them.


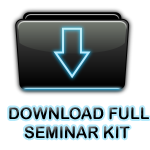
Need for large data processing
We live in the data age. It’s not easy to measure the total volume of data stored electronically, but an IDC estimate put the size of the “digital universe” at 0.18 zettabytes in 2006, and is forecasting a tenfold growth by 2011 to 1.8 zettabytes.
Some of the large data processing needed areas include:-
• The New York Stock Exchange generates about one terabyte of new trade data per day.
• Facebook hosts approximately 10 billion photos, taking up one petabyte of storage.
• Ancestry.com, the genealogy site, stores around 2.5 petabytes of data.
• The Internet Archive stores around 2 petabytes of data, and is growing at a rate of 20 terabytes per month.
• The Large Hadron Collider near Geneva, Switzerland, will produce about 15 petabytes of data per year.
The problem is that while the storage capacities of hard drives have increased massively over the years, access speeds—the rate at which data can be read from drives have not kept up. One typical drive from 1990 could store 1370 MB of data and had a transfer speed of 4.4 MB/s,§ so we could read all the data from a full drive in around five minutes. Almost 20 years later one terabyte drives are the norm, but the transfer speed is around 100 MB/s, so it takes more than two and a half hours to read all the data off the disk. This is a long time to read all data on a single drive—and writing is even slower. The obvious way to reduce the time is to read from multiple disks at once. Imagine if we had 100 drives, each holding one hundredth of the data. Working in parallel, we could read the data in under two minutes.This shows the significance of distributed computing.
Challenges in distributed computing --- meeting hadoop
Various challenges are faced while developing a distributed application. The first problem to solve is hardware failure: as soon as we start using many pieces of hardware, the chance that one will fail is fairly high. A common way of avoiding data loss is through replication: redundant copies of the data are kept by the system so that in the event of failure, there is another copy available. This is how RAID works, for instance, although Hadoop’s filesystem, the Hadoop Distributed Filesystem(HDFS), takes a slightly different approach.
The second problem is that most analysis tasks need to be able to combine the data in some way; data read from one disk may need to be combined with the data from any of the other 99 disks. Various distributed systems allow data to be combined from multiple sources, but doing this correctly is notoriously challenging. MapReduce provides a programming model that abstracts the problem from disk reads and writes transforming it into a computation over sets of keys and values.
This, in a nutshell, is what Hadoop provides: a reliable shared storage and analysis system. The storage is provided by HDFS, and analysis by MapReduce. There are other parts to Hadoop, but these capabilities are its kernel.
Hadoop is the popular open source implementation of MapReduce, a powerful tool designed for deep analysis and transformation of very large data sets. Hadoop enables you to explore complex data, using custom analyses tailored to your information and questions. Hadoop is the system that allows unstructured data to be distributed across hundreds or thousands of machines forming shared nothing clusters, and the execution of Map/Reduce routines to run on the data in that cluster. Hadoop has its own filesystem which replicates data to multiple nodes to ensure if one node holding data goes down, there are at least 2 other nodes from which to retrieve that piece of information. This protects the data availability from node failure, something which is critical when there are many nodes in a cluster (aka RAID at a server level).
COMPARISON WITH OTHER SYSTEMS
Comparison with RDBMS
Unless we are dealing with very large volumes of unstructured data (hundreds of GB, TB’s or PB’s) and have large numbers of machines available you will likely find the performance of Hadoop running a Map/Reduce query much slower than a comparable SQL query on a relational database. Hadoop uses a brute force access method whereas RDBMS’s have optimization methods for accessing data such as indexes and read-ahead. The benefits really do only come into play when the positive of mass parallelism is achieved, or the data is unstructured to the point where no RDBMS optimizations can be applied to help the performance of queries.
But with all benchmarks everything has to be taken into consideration. For example, if the data starts life in a text file in the file system (e.g. a log file) the cost associated with extracting that data from the text file and structuring it into a standard schema and loading it into the RDBMS has to be considered. And if you have to do that for 1000 or 10,000 log files that may take minutes or hours or days to do (with Hadoop you still have to copy the files to its file system). It may also be practically impossible to load such data into a RDBMS for some environments as data could be generated in such a volume that a load process into a RDBMS cannot keep up. So while using Hadoop your query time may be slower (speed improves with more nodes in the cluster) but potentially your access time to the data may be improved.
Also as there aren’t any mainstream RDBMS’s that scale to thousands of nodes, at some point the sheer mass of brute force processing power will outperform the optimized, but restricted on scale, relational access method. In our current RDBMS-dependent web stacks, scalability problems tend to hit the hardest at the database level. For applications with just a handful of common use cases that access a lot of the same data, distributed in-memory caches, such as memcached provide some relief. However, for interactive applications that hope to reliably scale and support vast amounts of IO, the traditional RDBMS setup isn’t going to cut it. Unlike small applications that can fit their most active data into memory, applications that sit on top of massive stores of shared content require a distributed solution if they hope to survive the long tail usage pattern commonly found on content-rich site. We can’t use databases with lots of disks to do large-scale batch analysis. This is because seek time is improving more slowly than transfer rate. Seeking is the process of moving the disk’s head to a particular place on the disk to read or write data. It characterizes the latency of a disk operation, whereas the transfer rate corresponds to a disk’s bandwidth. If the data access pattern is dominated by seeks, it will take longer to read or write large portions of the dataset than streaming through it, which operates at the transfer rate. On the other hand, for updating a small proportion of records in a database, a traditional B-Tree (the data structure used in relational databases, which is limited by the rate it can perform seeks) works well. For updating the majority of a database, a B-Tree is less efficient than MapReduce, which uses Sort/Merge to rebuild the database.
Another difference between MapReduce and an RDBMS is the amount of structure in the datasets that they operate on. Structured data is data that is organized into entities that have a defined format, such as XML documents or database tables that conform to a particular predefined schema. This is the realm of the RDBMS. Semi-structured data, on the other hand, is looser, and though there may be a schema, it is often ignored, so it may be used only as a guide to the structure of the data: for example, a spreadsheet, in which the structure is the grid of cells, although the cells themselves may hold any form of data. Unstructured data does not have any particular internal structure: for example, plain text or image data. MapReduce works well on unstructured or semistructured data, since it is designed to interpret the data at processing time. In otherwords, the input keys and values for MapReduce are not an intrinsic property of the data, but they are chosen by the person analyzing the data. Relational data is often normalized to retain its integrity, and remove redundancy. Normalization poses problems for MapReduce, since it makes reading a record a nonlocal operation, and one of the central assumptions that MapReduce makes is that it is possible to perform (high-speed) streaming reads and writes.
Traditional RDBMS | MapReduce | |
Data size | Gigabytes | Petabytes |
Access | Interactive and batch | Batch |
Updates | Read and write many times | Write once, read many times |
Structure | Static schema | Dynamic schema |
Integrity | High | Low |
Scaling | Non linear | Linear |
But hadoop hasn’t been much popular yet. MySQL and other RDBMS’s have stratospherically more market share than Hadoop, but like any investment, it’s the future you should be considering. The industry is trending towards distributed systems, and Hadoop is a major player.
ORIGIN OF HADOOP
Hadoop was created by Doug Cutting, the creator of Apache Lucene, the widely used text search library. Hadoop has its origins in Apache Nutch, an open source web searchengine, itself a part of the Lucene project. Building a web search engine from scratch was an ambitious goal, for not only is the software required to crawl and index websites complex to write, but it is also a challenge to run without a dedicated operations team, since there are so many moving parts. It’s expensive too: Mike Cafarella and Doug Cutting estimated a system supporting a 1-billion-page index would cost around half a million dollars in hardware, with a monthly running cost of $30,000.‖ Nevertheless, they believed it was a worthy goal, as it would open up and ultimately democratize search engine algorithms. Nutch was started in 2002, and a working crawler and search system quickly emerged. However, they realized that their architecture wouldn’t scale to the billions of pages on the Web. Help was at hand with the publication of a paper in 2003 that described the architecture of Google’s distributed filesystem, called GFS, which was being used in production at Google.# GFS, or something like it, would solve their storage needs for the very large files generated as a part of the web crawl and indexing process. In particular, GFS would free up time being spent on administrative tasks such as managing storage nodes. In 2004, they set about writing an open source implementation, the Nutch Distributed Filesystem (NDFS). In 2004, Google published the paper that introduced MapReduce to the world.* Early in 2005, the Nutch developers had a working MapReduce implementation in Nutch, and by the middle of that year all the major Nutch algorithms had been ported to run using MapReduce and NDFS. NDFS and the MapReduce implementation in Nutch were applicable beyond the realm of search, and in February 2006 they moved out of Nutch to form an independent subproject of Lucene called Hadoop. At around the same time, Doug Cutting joined Yahoo!, which provided a dedicated team and the resources to turn Hadoop into a system that ran at web scale (see sidebar). This was demonstrated in February 2008 when Yahoo! announced that its production search index was being generated by a 10,000-core Hadoop cluster. In April 2008, Hadoop broke a world record to become the fastest system to sort a terabyte of data. Running on a 910-node cluster, Hadoop sorted one terabyte in 2009 seconds (just under 3½ minutes), beating the previous year’s winner of 297 seconds(described in detail in “TeraByte Sort on Apache Hadoop” on page 461). In November of the same year, Google reported that its MapReduce implementation sorted one terabyte in 68 seconds.§ As this book was going to press (May 2009), it was announced that a team at Yahoo! used Hadoop to sort one terabyte in 62 seconds.
SUBPROJECTS
Although Hadoop is best known for MapReduce and its distributed filesystem(HDFS, renamed from NDFS), the other subprojects provide complementary services, or build on the core to add higher-level abstractions The various subprojects of hadoop includes:-
Core
A set of components and interfaces for distributed filesystems and general I/O(serialization, Java RPC, persistent data structures).
Avro
A data serialization system for efficient, cross-language RPC, and persistent datastorage. (At the time of this writing, Avro had been created only as a new subproject, and no other Hadoop subprojects were using it yet.)
Mapreduce
A distributed data processing model and execution environment that runs on large clusters of commodity machines.
HDFS
A distributed filesystem that runs on large clusters of commodity machines.
Pig
A data flow language and execution environment for exploring very large datasets. Pig runs on HDFS and MapReduce clusters.
HBASE
A distributed, column-oriented database. HBase uses HDFS for its underlying storage, and supports both batch-style computations using MapReduce and point queries (random reads).
Zookeeper
A distributed, highly available coordination service. ZooKeeper provides primitives such as distributed locks that can be used for building distributed applications.
Hive
A distributed data warehouse. Hive manages data stored in HDFS and provides a query language based on SQL (and which is translated by the runtime engine to MapReduce jobs) for querying the data.
Chukwa
A distributed data collection and analysis system. Chukwa runs collectors that store data in HDFS, and it uses MapReduce to produce reports. (At the time of this writing, Chukwa had only recently graduated from a “contrib” module in Core to its own subproject.)
THE HADOOP APPROACH
Hadoop is designed to efficiently process large volumes of information by connecting many commodity computers together to work in parallel. The theoretical 1000-CPU machine described earlier would cost a very large amount of money, far more than 1,000 single-CPU or 250 quad-core machines. Hadoop will tie these smaller and more reasonably priced machines together into a single cost-effective compute cluster.
Performing computation on large volumes of data has been done before, usually in a distributed setting. What makes Hadoop unique is its simplified programming model which allows the user to quickly write and test distributed systems, and its efficient, automatic distribution of data and work across machines and in turn utilizing the underlying parallelism of the CPU cores.
Data distribution
In a Hadoop cluster, data is distributed to all the nodes of the cluster as it is being loaded in. The Hadoop Distributed File System (HDFS) will split large data files into chunks which are managed by different nodes in the cluster. In addition to this each chunk is replicated across several machines, so that a single machine failure does not result in any data being unavailable. An active monitoring system then re-replicates the data in response to system failures which can result in partial storage. Even though the file chunks are replicated and distributed across several machines, they form a single namespace, so their contents are universally accessible.
Data is conceptually record-oriented in the Hadoop programming framework. Individual input files are broken into lines or into other formats specific to the application logic. Each process running on a node in the cluster then processes a subset of these records. The Hadoop framework then schedules these processes in proximity to the location of data/records using knowledge from the distributed file system. Since files are spread across the distributed file system as chunks, each compute process running on a node operates on a subset of the data. Which data operated on by a node is chosen based on its locality to the node: most data is read from the local disk straight into the CPU, alleviating strain on network bandwidth and preventing unnecessary network transfers. This strategy of moving computation to the data, instead of moving the data to the computation allows Hadoop to achieve high data locality which in turn results in high performance.
MapReduce: Isolated Processes
Hadoop limits the amount of communication which can be performed by the processes, as each individual record is processed by a task in isolation from one another. While this sounds like a major limitation at first, it makes the whole framework much more reliable. Hadoop will not run just any program and distribute it across a cluster. Programs must be written to conform to a particular programming model, named "MapReduce."
In MapReduce, records are processed in isolation by tasks called Mappers. The output from the Mappers is then brought together into a second set of tasks called Reducers, where results from different mappers can be merged together.
Separate nodes in a Hadoop cluster still communicate with one another. However, in contrast to more conventional distributed systems where application developers explicitly marshal byte streams from node to node over sockets or through MPI buffers, communication in Hadoop is performed implicitly. Pieces of data can be tagged with key names which inform Hadoop how to send related bits of information to a common destination node. Hadoop internally manages all of the data transfer and cluster topology issues.
By restricting the communication between nodes, Hadoop makes the distributed system much more reliable. Individual node failures can be worked around by restarting tasks on other machines. Since user-level tasks do not communicate explicitly with one another, no messages need to be exchanged by user programs, nor do nodes need to roll back to pre-arranged checkpoints to partially restart the computation. The other workers continue to operate as though nothing went wrong, leaving the challenging aspects of partially restarting the program to the underlying Hadoop layer.
INTRODUCTION TO MAPREDUCE
MapReduce is a programming model and an associated implementation for processing and generating largedata sets. Users specify a map function that processes a key/value pair to generate a set of intermediate key/value pairs, and a reduce function that merges all intermediate values associated with the same intermediate key. Many real world tasks are expressible in this model.
This abstraction is inspired by the map and reduce primitives present in Lisp and many other functional languages. We realized that most of our computations involved applying a map operation to each logical .record. in our input in order to compute a set of intermediate key/value pairs, and then applying a reduce operation to all the values that shared the same key, in order to combine the derived data appropriately. Our use of a functional model with user specilized map and reduce operations allows us to parallelize large computations easily and to use re-execution as the primary mechanism for fault tolerance.
Programming model
The computation takes a set of input key/value pairs, and produces a set of output key/value pairs. The user of the MapReduce library expresses the computation as two functions: Map and Reduce. Map, written by the user, takes an input pair and produces a set of intermediate key/value pairs. The MapReduce library groups together all intermediate values associatedwith the same intermediate key I and passes them to the Reduce function. The Reduce function, also written by the user, accepts an intermediate key I and a set of values for that key. It merges together these values to form a possibly smaller set of values. Typically just zero or one output value is produced per Reduce invocation. The intermediate values are supplied to the user's reduce function via an iterator. This allows us to handle lists of values that are too large to fit in memory.
Example: Sum Reducer
let reduce(k, vals)
sum = 0
foreach int v in vals:
sum += v
emit(k, sum)
(“A”, [42, 100, 312]) --> (“A”, 454)
(“B”, [12, 6, -2]) --> (“B”, 16)
Example2:-
Counting the number of occurrences of each word in a large collection of documents. The user would write code similar to the following pseudo-code:
map(String key, String value):
// key: document name
// value: document contents
for each word w in value:
EmitIntermediate(w, "1");
reduce(String key, Iterator values):
// key: a word
// values: a list of counts
int result = 0;
for each v in values:
result += ParseInt(v);
Emit(AsString(result));
The map function emits each word plus an associated count of occurrences (just `1' in this simple example). The reduce function sums together all counts emitted for a particular word.
In addition, the user writes code to _ll in a mapreduce specification object with the names of the input and output _les, and optional tuning parameters. The user then invokes the MapReduce function, passing it the specification object. The user's code is linked together with the MapReduce library (implemented in C++)
Programs written in this functional style are automatically parallelized and executed on a large cluster of commodity machines. The run-time system takes care of the details of partitioning the input data, scheduling the program's execution across a set of machines, handling machine failures, and managing the required inter-machine communication. This allows programmers without any experience with parallel and distributed systems to easily utilize the resources of a large distributed system.
The issues of how to parallelize the computation, distribute the data, and handle failures conspire to obscure the original simple computation with large amounts of complex code to deal with these issues. As a reaction to this complexity, Google designed a new abstraction that allows us to express the simple computations we were trying to perform but hides the messy details of parallelization, fault-tolerance, data distribution and load balancing in a library.
Types
Even though the previous pseudo-code is written in terms of string inputs and outputs, conceptually the map and reduce functions supplied by the user have associated
types:
map (k1,v1) ! list(k2,v2)
reduce (k2,list(v2)) ! list(v2)
I.e., the input keys and values are drawn from a different domain than the output keys and values. Furthermore, the intermediate keys and values are from the same domain as the output keys and values. Our C++ implementation passes strings to and from the user-de_ned functions and leaves it to the user code to convert between strings and appropriate types.
Inverted Index: The map function parses each document, and emits a sequence of hword; document IDi pairs. The reduce function accepts all pairs for a given word, sorts the corresponding document IDs and emits a hword; list(document ID)i pair. The set of all output pairs forms a simple inverted index. It is easy to augment this computation to keep track of word positions.
Distributed Sort: The map function extracts the key from each record, and emits a hkey; recordi pair. The reduce function emits all pairs unchanged.
HADOOP MAPREDUCE
Hadoop Map-Reduce is a software framework for easily writing applications which process vast amounts of data (multi-terabyte data-sets) in-parallel on large clusters (thousands of nodes) of commodity hardware in a reliable, fault-tolerant manner.
A Map-Reduce job usually splits the input data-set into independent chunks which are processed by the map tasks in a completely parallel manner. The framework sorts the outputs of the maps, which are then input to the reduce tasks. Typically both the input and the output of the job are stored in a file-system. The framework takes care of scheduling tasks, monitoring them and re-executes the failed tasks.
Typically the compute nodes and the storage nodes are the same, that is, the Map-Reduce framework and the Distributed FileSystem are running on the same set of nodes. This configuration allows the framework to effectively schedule tasks on the nodes where data is already present, resulting in very high aggregate bandwidth across the cluster.
A MapReduce job is a unit of work that the client wants to be performed: it consists of the input data, the MapReduce program, and configuration information. Hadoop runs the job by dividing it into tasks, of which there are two types: map tasks and reduce tasks. There are two types of nodes that control the job execution process: a jobtracker and a number of tasktrackers. The jobtracker coordinates all the jobs run on the system by scheduling tasks to run on tasktrackers. Tasktrackers run tasks and send progress reports to the jobtracker, which keeps a record of the overall progress of each job. If a tasks fails, the jobtracker can reschedule it on a different tasktracker. Hadoop divides the input to a MapReduce job into fixed-size pieces called input splits, or just splits. Hadoop creates one map task for each split, which runs the userdefined map function for each record in the split.
Having many splits means the time taken to process each split is small compared to the time to process the whole input. So if we are processing the splits in parallel, the processing is better load-balanced if the splits are small, since a faster machine will be able to process proportionally more splits over the course of the job than a slower machine. Even if the machines are identical, failed processes or other jobs running concurrently make load balancing desirable, and the quality of the load balancing increases as the splits become more fine-grained. On the other hand, if splits are too small, then the overhead of managing the splits and of map task creation begins to dominate the total job execution time. For most jobs, a good split size tends to be the size of a HDFS block, 64 MB by default, although this can be changed for the cluster (for all newly created files), or specified when each file is created. Hadoop does its best to run the map task on a node where the input data resides in HDFS. This is called the data locality optimization. It should now be clear why the optimal split size is the same as the block size: it is the largest size of input that can be guaranteed to be stored on a single node. If the split spanned two blocks, it would be unlikely that any HDFS node stored both blocks, so some of the split would have to be transferred across the network to the node running the map task, which is clearly less efficient than running the whole map task using local data. Map tasks write their output to local disk, not to HDFS. Map output is intermediate output: it’s processed by reduce tasks to produce the final output, and once the job is complete the map output can be thrown away. So storing it in HDFS, with replication, would be overkill. If the node running the map task fails before the map output has been consumed by the reduce task, then Hadoop will automatically rerun the map task on another node to recreate the map output. Reduce tasks don’t have the advantage of data locality—the input to a single reduce task is normally the output from all mappers. In the present example, we have a single reduce task that is fed by all of the map tasks. Therefore the sorted map outputs have to be transferred across the network to the node where the reduce task is running, where they are merged and then passed to the user-defined reduce function. The output of the reduce is normally stored in HDFS for reliability. For each HDFS block of the reduce output, the first replica is stored on the local node, with other replicas being stored on off-rack nodes. Thus, writing the reduce output does consume network bandwidth, but only as much as a normal HDFS write pipeline consume. The dotted boxes in the figure below indicate nodes, the light arrows show data transfers on a node, and the heavy arrows show data transfers between nodes. The number of reduce tasks is not governed by the size of the input, but is specified independently.
When there are multiple reducers, the map tasks partition their output, each creating one partition for each reduce task. There can be many keys (and their associated values) in each partition, but the records for every key are all in a single partition. The partitioning can be controlled by a user-defined partitioning function, but normally the default partitioner—which buckets keys using a hash function—works very well. This diagram makes it clear why the data flow between map and reduce tasks is colloquially known as “the shuffle,” as each reduce task is fed by many map tasks. The shuffle is more complicated than this diagram suggests, and tuning it can have a big impact on job execution time. Finally, it’s also possible to have zero reduce tasks. This can be appropriate when you don’t need the shuffle since the processing can be carried out entirely in parallel.
Combiner Functions
Many MapReduce jobs are limited by the bandwidth available on the cluster, so it pays to minimize the data transferred between map and reduce tasks. Hadoop allows the user to specify a combiner function to be run on the map output—the combiner function’s output forms the input to the reduce function. Since the combiner function is an optimization, Hadoop does not provide a guarantee of how many times it will call it for a particular map output record, if at all. In other words, calling the combiner function zero, one, or many times should produce the same output from the reducer.
< FULL REPORT CAN DOWNLOAD, ITS NOT FULL>
No comments:
Post a Comment
leave your opinion